Speakers
Aleyda Solis – Founder & International SEO Consultant at Orainti
Rick Viscomi – Core Web Vitals DevRel at Google
JR Oakes – VP of Strategy at LOCOMOTIVE Agency
Paul Shapiro – Web Intelligence at Uber
Kevin Indig – Growth Advisor for Hims, Dropbox, Reddit, and more
Rachel Anderson – Senior SEO Manager at Weedmaps
Fili Wiese – SEO Consultant, Ex Google Engineer
Michael King – Founder & Chief Executive Officer at iPullRank
Kristin Tynski – Co-Founder and SVP of Creative at Fractl
Noah Learner – Director of Innovation at Sterling Sky
Sam Torres – Chief Digital Officer at Gray Dot Company
Rick Viscomi – Core Web Vitals DevRel at Google
Dan Hinckley – Chief Technical Officer and Co-Founder at Go Fish Digital
Jori Ford – CMO at FoodBoss
Lazarina Stoy – Founder at MLOpsSEO and Consultant
Fabrice Canel – Bing Product Manager at Microsoft
Victor Pan – Principal SEO at HubSpot
Serge Bezborodov – CTO at JetOctopus
Patrick Stox – Product Advisor at Ahrefs

TECH SEO CONNECT 2024, Raleigh, NC - My Notes
Summary Highlights:
-
The Fast Pace of Change in SEO: The evolution of AI, particularly language models (LLMs), is impacting SEO, creating both challenges (e.g., uncertain search interfaces, content reliability) and opportunities (e.g., automation in SEO tasks).
-
Rise of Automation Tools: Technical SEOs now have a variety of automation tools for tasks like internal linking, structured data implementation, and knowledge graph development. Tools like ChatGPT, Screaming Frog, and cloud-based crawlers allow for more advanced, scalable automation.
-
Challenges of Implementation: While there’s plenty of tech available for technical SEO analysis and auditing, the real challenge remains in implementation. Many SEOs struggle to get their recommendations executed—only 40-60% of technical recommendations are typically implemented.
-
Human Factors Matter: Technical SEOs need stakeholder buy-in and better internal communication to fully implement SEO changes. This is where automation can’t help—human interaction and prioritization are essential.
-
Popular Tools in Use: Google Search Console, Screaming Frog, and SEOMRush remain the most popular tools for SEO audits and analysis. However, the industry is evolving with more integration and automation tools being widely adopted.
-
Forecasting and Prioritization: Simple Machine Learning for Sheets is a useful, free forecasting tool that integrates with Google Search Console data. SEOs need to focus more on forecasting traffic and revenue impact to secure decision-maker buy-in.
-
Ongoing Challenges: Despite new tools and automation, the SEO industry still faces long-standing issues like resource constraints, JavaScript rendering, and communication between SEOs, developers, and stakeholders.
Action Items:
-
Leverage Automation Tools: Explore tools like Screaming Frog and ChatGPT for automating repetitive technical tasks like internal linking and structured data.
-
Stakeholder Buy-In: Use tools like Jira and Slack to align with product teams and developers. Develop clear, actionable user stories to communicate SEO recommendations and track execution.
-
Enhance Forecasting: Implement forecasting models like ARIMA or ETS with tools like Simple ML for Sheets. Use forecasts to estimate traffic and revenue impacts to convince decision-makers.
-
Prioritize Implementation: Use the RICE prioritization model (Reach, Impact, Confidence, Effort) to ensure technical SEO recommendations with the most impact are prioritized.
-
Improve Communication: Develop tailored SEO reports that highlight the value of technical SEO changes in terms that resonate with decision-makers, emphasizing the business impact and clear KPIs.
Optimizing Core Web Vitals

Summary Highlights:
1. Interaction to Next Paint (INP) Launch: Rick mentioned the successful transition from First Input Delay (FID) to INP as a core web vital metric. Thanks to the SEO community’s awareness efforts, INP pass rates increased from 65% to 76% on mobile after the launch.
2. Value Beyond SEO: Improving Core Web Vitals benefits not just SEO rankings but also user experience, business metrics (e.g., bounce rate, conversion rate), and engagement. Examples like YouTube’s Project Feather and Sunday Citizen's improvements show how even small web performance tweaks can impact users globally.
3. Largest Contentful Paint (LCP): This remains one of the most challenging metrics for many websites. Rick highlighted the need to focus on discoverability (e.g., avoiding background images for LCP, using proper HTML image tags) and ensuring critical elements are not lazily loaded or deprioritized.
4. Cumulative Layout Shift (CLS): He emphasized reserving space for content to prevent shifts, using aspect ratios in CSS, and leveraging modern techniques like BFCache (Back-Forward Cache) to enhance layout stability.
5. JavaScript & CSS Optimization: Rick discussed the importance of reducing unnecessary JavaScript, yielding long tasks, and avoiding layout thrashing. These practices help reduce rendering delays and improve interaction performance.
6. Real User Measurement (RUM) vs. Lab Data: While lab tools like Lighthouse are helpful, real user measurement (RUM) provides more accurate insights into actual user experiences. He showcased how RUM data helped diagnose INP issues on the web.dev site.
Action Items:
1. Improve LCP by Prioritizing Critical Resources: Ensure critical images are discoverable in HTML, not hidden in CSS or JavaScript.Avoid using loading="lazy" for critical content and use fetchpriority="high" to prioritize key resources.
2. Leverage BFCache: Utilize the Back-Forward Cache to deliver near-instant navigations on mobile, improving user experiences significantly, especially for repeat visitors.
3. Use a CDN for Faster Delivery: Adopt CDNs to distribute content closer to users and enable compression, caching, and advanced protocols.
4. Optimize CLS by Reserving Space: Explicitly set dimensions for images, videos, and other visual elements using the aspect-ratio property in CSS to minimize layout shifts.
5. Optimize JavaScript Execution: Remove or defer non-critical JavaScript. Break long tasks into smaller chunks using yielding methods like setTimeout or the scheduler.postTask() API.
6. Adopt Real User Monitoring (RUM): Implement RUM solutions to collect real-time performance data from actual users, helping diagnose and improve issues related to INP, LCP, and CLS.
7. Focus on Business Impact: Track improvements in core web vitals alongside business metrics like conversion rates, bounce rates, and engagement to showcase the tangible benefits of better performance.
These insights and actions from Rick’s session will help optimize Core Web Vitals effectively, enhancing both SEO and overall user experience.
Npath Leveraging LLMs to Extract Insights from GA4 Event Data
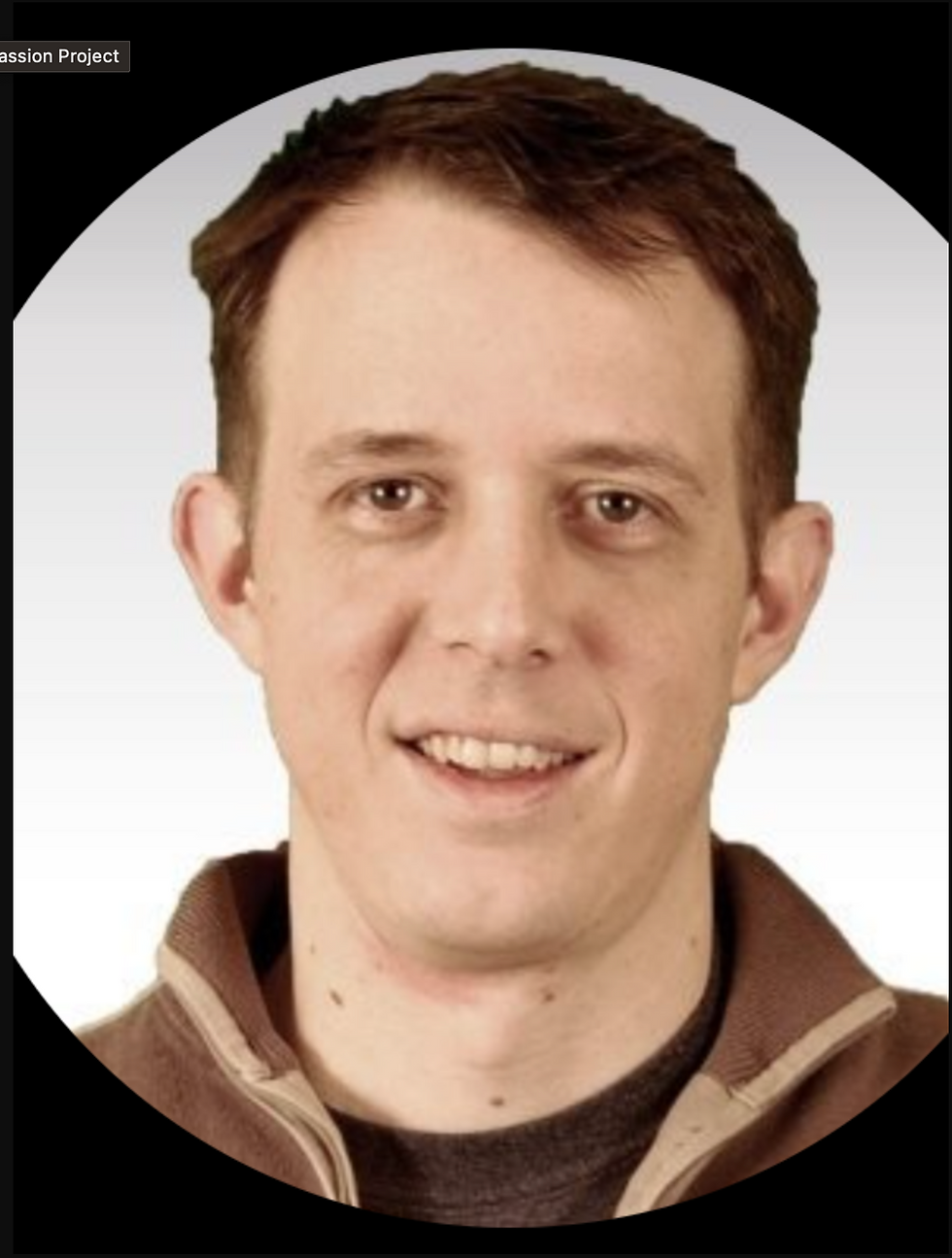
Summary Highlights:
-
LLMs & Unlimited Context Windows: Oakes discussed how language models (LLMs) with large context windows (up to millions of tokens) can help transform how we analyze data, especially in extracting insights from GA4 event data. These models can process vast amounts of information (like whole books) and retrieve hidden patterns.
-
Sequence Analysis on Event Data: Oakes introduced Empath, a tool that uses genetic algorithm-like sequence analysis to study event data from Google Analytics. This allows SEOs to analyze user behavior patterns, paths, and cohorts, providing insights on user preferences and next-page behaviors.
-
NPath Overview: NPath is a project that automates the analysis of URLs by regularly crawling pages and comparing data changes over time. By feeding GA4, Search Console, and other datasets into LLMs, NPath can send automated alerts about significant changes in traffic, page performance, or user flow. It’s designed to make it easier for SEOs to track performance without manual deep-diving.
-
Open Source & Customizable: Oakes emphasized that NPath is open-source, allowing users to customize prompts, analysis criteria, and data integrations (e.g., PageSpeed Insights, SERP performance, etc.).
-
Real-Time Alerts: One key feature of NPath is the ability to set thresholds for important changes in performance, which triggers email notifications with analysis results. It helps in tracking user behavior, next-page drops, and optimization efforts without constant manual checks.
-
Use of LLMs for Data Insights: Oakes highlighted how LLMs can transform complex event data into actionable insights, offering a method to analyze paths, identify anomalies, and extract behavioral trends from users' journey on a website.
Action Items:
-
Integrate Empath for Event Data Analysis:Utilize Empath to analyze event sequences in GA4. This can help identify key user flows, behavioral cohorts, and predict next actions based on prior events.
-
Set Up NPath for Automated Insights:Set up NPath to crawl your site, GA4 event data, and Search Console at regular intervals. Leverage it to automatically alert you to significant changes in user paths, page performance, or traffic drops.
-
Customize Prompts & Analysis Criteria:Modify NPath’s prompts to suit specific business needs. This allows for tailored insights based on the thresholds and key metrics that matter most to your SEO efforts.
-
Use LLMs for Anomaly Detection: Implement LLMs to detect and analyze anomalies in GA4 event data, like sudden drops in conversions or unusual traffic patterns, which can guide your optimization strategies.
-
Monitor Next Page Analysis & Pathing: Use the insights from NPath to improve next-page paths and address pages with declining user engagement after critical touchpoints (e.g., homepage to contact form).
Getting Technical SEO Work Done at Fortune 100 Companies

Summary Highlights:
1. The SEO "Ship Show": Indig emphasized that technical SEO work is meaningless if recommendations aren't implemented. Most SEO professionals struggle with getting their suggestions shipped—less than 50% of recommendations typically get executed. This presentation focused on strategies to ensure technical SEO work actually gets shipped.
2. Story-Based Pitching Over Data-Driven Pitches: While data is essential, storytelling resonates more with executives. Indig shared how at Shopify, precise data often led to discussions that delayed action. Story-based pitches, tied to company goals and context, resulted in quicker execution.
Key takeaway: Executives are driven by emotions and instincts more than just numbers. Tie your recommendations to the company’s specific situation (e.g., turnaround, growth acceleration) and make them align with high-level business goals (revenue, market share, risk).
3. Starting with the Goal, Not the Details: Presentations should focus on three key priorities, making it easier for executives to decide. Extensive, detail-heavy audits often overwhelm leadership and cause decision fatigue.
Key takeaway: Always keep recommendations focused on high-level goals, how they impact business outcomes, and avoid overwhelming stakeholders with excessive details.
4. Deep vs. Shallow Recommendations: Shallow recommendations like “fix international indexation” don’t provide actionable steps for implementation. Instead, providing deep recommendations that outline specific tasks and subtasks, with clear context and expected outcomes, will get you better results.
Key takeaway: Make your recommendations detailed and actionable. Describe the ideal outcome and give clear steps for engineers or stakeholders to follow.
5. Three Common Mistakes in Leadership Pitches:
- Too many unnecessary details
- Unsupported big claims that lose trust
- Doubting yourself in delivery
6. Focus on Risk, Not Just Rewards: Leaders care more about minimizing risk than maximizing upside. Therefore, presenting what's at stake if changes are not made is a critical part of getting buy-in.
7. Back Channeling and Personalized Communication: Meet with key decision-makers one-on-one before big presentations to gather feedback and address hesitations in advance. Use their wording in your presentations to make them feel part of the solution.
8. Clarity in Communication: To get things implemented, you need to be very clear with the root cause of the problem, the specific tasks to solve it, and the ideal outcome. Indig stressed the importance of clarity when communicating with engineering teams or other departments.
Action Items:
1. Adopt Story-Based Pitching:
- Tie your technical SEO recommendations to the broader business context (e.g., growth acceleration, turnaround), and emphasize the high-level outcomes they support (e.g., market share, profit, risk reduction).
2. Focus on 3 Main Recommendations:
- Prioritize three key takeaways in your presentations. Avoid overwhelming decision-makers with excessive technical details; stick to what will move the needle and how it impacts business goals.
3. Make Recommendations Actionable:
- For every recommendation, break it down into actionable tasks and subtasks. Define clear outcomes so engineering teams know exactly what to do without needing additional discovery work.
4. Highlight Risks Alongside Rewards:
- Always explain the potential consequences if the recommendation isn’t implemented. Highlighting the stakes will help get leadership buy-in faster.
5. Improve Personalization in Communication:
- Meet individually with key stakeholders to gather feedback before presenting to a larger audience. Use their language and align your recommendations with their specific goals to increase buy-in.
Gaining Velocity: Building SEO into a Continuous Development Process

Summary Highlights:
1. Tech Debt vs. New Features: Rachel highlighted a common issue where resources are heavily consumed by technical debt, preventing progress on new SEO features. At Weedmaps, the engineering team was spending a third of their sprint points on tech debt, leading to delays and issues in new initiatives.
2. Reactive vs. Proactive Approach: The core issue was a reactive approach to SEO problems—bugs and regressions were already impacting search rankings by the time they were discovered. The team relied on tools like Sitebulb and GSC but needed a more proactive strategy to catch errors before they affected search engine indexing.
3. Proactive Four-Pronged Solution:
- Automated Tests in CI/CD Pipeline: Incorporating SEO tests into the continuous integration/continuous delivery (CI/CD) pipeline to catch issues before they go live. Rachel’s team worked with the QE team to configure tests specific to SEO needs, including regionalized tests for the heavily localized cannabis market.
- SEO Sign-Off in Business Processes: Introducing SEO sign-off at key phases of project development, particularly in the Product Requirements Document (PRD) phase. This included adding a Googlebot user story and acceptance criteria to ensure SEO elements like canonical tags, headers, and filters weren’t overlooked or incorrectly implemented.
- SEO QA for High-Risk Tickets: Implementing a tagging system in Jira where tickets that could impact SEO are flagged for SEO review. If these tickets are not reviewed, they are blocked from deployment. This helped ensure that no changes went live without proper SEO consideration.
- Engineering Education: Educating engineering teams on SEO fundamentals (crawl, render, index) and providing accessible documentation in their internal wikis. This helped engineers proactively think about SEO impact when working on tickets.
4. Key Outcomes:
- Reduction in SEO Regression Bugs: By implementing these proactive measures, high-impact SEO bugs were cut in half.
- Improved Cross-Team Collaboration: The process improved communication between SEO, engineering, product, design, and legal teams, fostering a more collaborative environment.
- Faster Project Execution: The shift allowed teams to focus more on new feature development rather than constantly revisiting SEO issues.
5. Unexpected Benefits:
- Cross-Functional Collaboration: Teams across the organization (product, design, legal) improved their workflows and collaboration as a result of the updated business processes.
- Education at All Levels: Beyond engineers, educating product managers and business teams on SEO's role in website performance also helped align everyone on SEO goals.
Action Items:
1. Automate SEO Tests in Your CI/CD Pipeline:
- Work with QE and engineering teams to set up automated tests that catch SEO issues before code is pushed live. Customize tests for specific SEO concerns (e.g., canonical tags, H1 tags, metadata) and include regional checks if your site operates in localized markets.
2. Implement SEO Sign-Off in Business Processes:
- Introduce SEO review checkpoints in project phases like the PRD to ensure that SEO requirements are considered early in the process. Make sure SEO guidelines, such as Googlebot user stories, are embedded in every web-based project.
3. Set Up SEO QA for High-Risk Tickets:
- Use project management tools like Jira to flag tickets that may impact SEO, ensuring they are reviewed before going live. Create a dashboard to monitor these tickets and prevent overlooked SEO issues from reaching production.
4. Educate Engineers and Teams on SEO:
- Run workshops and provide accessible documentation for engineers, product managers, and other stakeholders to help them understand the critical role SEO plays in development. Focus on SEO fundamentals, regional challenges, and the specific impact of their work on search performance.
Improving SEO in Cloud Environments
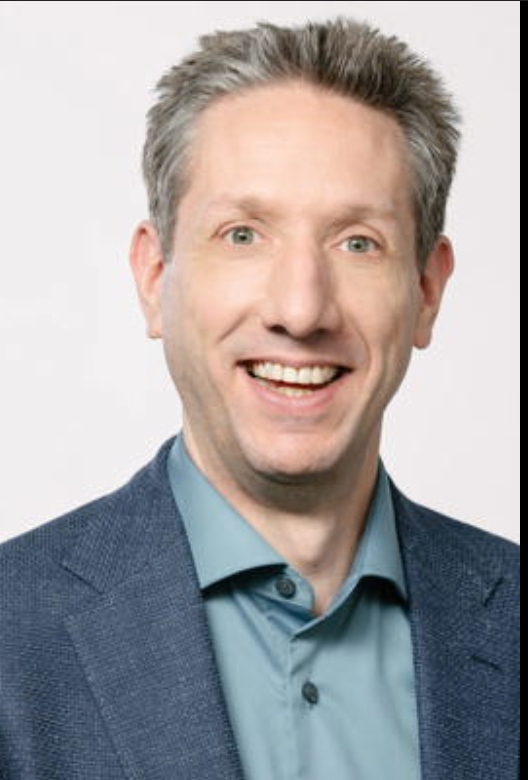
Summary Highlights:
1. Duplicate Content and URLs: 60% of the web is duplicate content, according to Google, and cloud environments can exacerbate this.
URL management is critical: treat URLs like database row IDs (immutable) and avoid unnecessary URL changes.
Staging environments, development servers, and CDN URLs can lead to content duplication that competes in search results.
2. Hostnames and Subdomains: Regularly check hostnames and subdomains for duplication, especially in cloud environments where external subdomains like staging sites, CDN links, or load balancer URLs might be indexed unintentionally.
Example: Walmart’s staging subdomains were indexable, which shouldn’t happen.
3. Logging and Server Data:
- Log data must be accurate: Ensure full URLs, including protocols (HTTP vs. HTTPS) and subdomain distinctions, are recorded in server logs.
- Incomplete logging means you can't fully track user requests, leading to poor SEO decisions.
4. Blocking and Allowing Bots: Some websites aggressively block bots with firewalls, which can unintentionally block Googlebot. Use Google’s list of IP addresses, but regularly update them to avoid issues.
CAPTCHAs and Cloudflare challenges can create SEO issues, generating infinite URLs and incorrectly returning 200 status codes.
5. Canonical and CDN Duplication Issues: CDN services, cloud storage (AWS S3, Google Cloud Storage), and other cloud-based infrastructure can duplicate content. Issues like misconfigured canonical tags on CDNs can lead to improper indexing (as seen with NASA and Goldman Sachs examples).
6. Serverless and Cloud Deployment Issues: Using cloud environments (like Google Cloud Run) can lead to unexpected URL duplications (e.g., load balancer URLs and low-level subdomains being indexed). You may need to hard-code canonical rules and redirects for cloud environments to avoid duplication and indexing issues.
7. Backend Optimization:
- Optimize backend resources (CPU, memory, disk, etc.) for improved speed and stability.
- Time to First Byte (TTFB): Slow TTFB signals poor backend optimization, potentially hurting your SEO.
- Cold start times in serverless environments can hurt performance, so always keep a minimum instance running.
8. Cloud Services and Speed:
- Tools like Google Search Console can help identify latency and performance issues, but direct cloud monitoring is essential for identifying resource bottlenecks.
- Content Delivery Networks (CDNs): Ensure compression protocols match across your CDN and origin servers to avoid rendering issues.
9. Database and Query Optimization:
- Reduce database queries and simplify SQL queries to improve performance.
- Caching and in-memory processing (like Redis or Memcached) can significantly improve website response times.
10. Cacheable Resources: Make sure all resources are cacheable and don’t use headers like "no-store" on cacheable assets.
11. Monitoring and Alerts: Set up alerts for status code errors (especially 500 errors) and unusual bot behavior to catch issues early.
- Use Google Search Console for logging bucket URLs, CDNs, and additional subdomains to monitor external duplication issues.
Takeaways:
- Prevent duplication: Regularly audit for off-domain content and take steps to block or redirect it.
- Fix backend issues: Optimize server resources, use appropriate caching, and eliminate bottlenecks to enhance speed and SEO performance.
- Be proactive with cloud and server-side issues by setting up proper logging, monitoring, and URL management to avoid potential SEO pitfalls.
Fili emphasized the complexity and importance of addressing content duplication, cloud infrastructure challenges, and backend optimization to ensure a robust SEO strategy in a cloud-based environment.
Video: https://www.youtube.com/watch?v=ObIItX0N2WM
Rolling Your Own Rank Tracking Solution
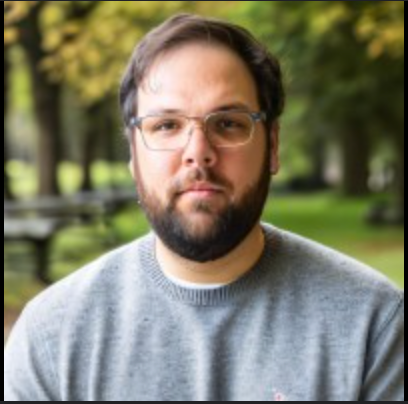
Summary Highlights:
-
Problem with Most Rank Tracking Solutions
- Lack of Flexibility: Many existing platforms lock users into limited features with little room for custom development.
- Siloed Data: Data often exists in separate silos, making it challenging to integrate rank tracking with other analytics tools.
2. Benefits of Building Your Own Rank Tracking Solution
- Full Control Over Data: All data is available within one system, giving more flexibility for analysis and customization.
- Customizable Features: If a feature is missing, it can be added without the need to switch providers.
- Open Source: Rank and Berry is an open-source project, enabling collaboration and customization by the community.
3. Technical Architecture of Rank and Berry
- Backend: Built using Python and FastAPI, a modern web framework that facilitates the creation of APIs and backends efficiently.
- Frontend: Built using Vue.js, a JavaScript framework for building modern, modular user interfaces.
- Database: Uses SQLite for local development but plans to move to PostgreSQL for more robust, multi-user applications.
- APIs Used:
- Space Serp (for rank tracking data)
- Google Search Console API (for click, impressions, and CTR data)
- Grepwords (for search volume data)
Core Features of Rank and Berry
1. Rank Tracking Data:
- Pulls rank data for keywords and domains.
- Displays keyword ranking, rank changes, search volume, and estimated business impact.
2. Scheduling & On-Demand Fetching:
- Automates the data-fetching process, allowing scheduled pulls or manual fetching of new data.
3. Tag Management System:
- Helps categorize and analyze data across different projects or domains, offering summary views by tags.
4. Google Search Console Integration:
- Gathers GSC data (clicks, impressions, CTR) to support deeper analysis.
5. Estimated Business Impact:
- Calculates the business value of ranking changes using GSC’s click-through rate data, user-defined conversion rates, and average conversion values.
6. Time Series & Share of Voice Analysis:
- Visualizes the ranking progress over time and provides share of voice insights across the top 10 search results.
Challenges and Limitations
-
Limited to One Rank Tracking API: Currently relies on Space Serp API, which lacks some advanced features (e.g., featured snippets, AI overviews).
-
SQLite as the Database: Not suitable for multi-user production environments, making it a future target for upgrading to PostgreSQL.
-
Missing Features: Due to time constraints, features like anomaly detection, algorithm update overlays, and forecasting are still on the roadmap.
-
Future Features and Improvements
-
Algorithm Update Overlays: Overlay algorithm updates on ranking data for better insights into sudden ranking changes.
-
Anomaly Detection: Detect unusual ranking changes automatically.
-
Forecasting: Use time series data to predict future rankings and revenue impact.
-
Keyword Clustering: Group keywords into clusters based on thematic similarity to better manage large keyword sets.
-
User Authentication: Move towards multi-user support by introducing user management and authentication systems.
Open Source and Contributions
- Community Contribution: Encouraged open-source contributions to the project to expand its capabilities, squash bugs, and add features.
- AGPL License: Rank and Berry uses a strong copyleft license to ensure that any modifications are shared back to the community.
In summary, Rank and Berry offers a customizable, open-source solution for those who want full control over their rank tracking data. It's designed for users looking to go beyond what existing platforms offer, allowing integration with other data sources and custom analysis. However, it is still evolving, with several important features planned for future development.
Video:
Chasing the Googlebot Trail

Summary Highlights:
Importance of Crawling in SEO
-
Crawling is fundamental: Jori stresses that crawling is the most critical part of SEO, as it determines whether Google can access and understand your website's content.
-
Crawl Stats and Tools: Google Search Console provides crawl stats, but Jori discusses how to go beyond these tools to gain more insights into Googlebot's behavior.
Crawl Stats in Google Search Console
Jori reviews various crawl stats available in GSC and how to interpret them for SEO purposes:
-
Total Crawl Requests: Not necessarily better with higher numbers; it depends on the site's size and indexing goals.
-
Download Size: Not always crucial unless your crawl budget is being used inefficiently due to large page sizes.
-
Average Response Time: Misleading when using server-side metrics alone; Jori suggests using DOM Content Load as a proxy for accurate response times.
-
Host Status: Only helpful when major issues arise, but Jori advises not to rely solely on this metric since GSC only flags problems after 90 days.
Identifying Crawl Gaps and Indexing Issues
-
Jori illustrates how to identify indexation gaps, showing a client case study where only part of the sitemap URLs were indexed, revealing a gap of 80,000 URLs.
-
Indexing Timeframes: Using tools to project the estimated time for full indexing can help set realistic expectations for stakeholders.
Mapping Googlebot Entry Points and Site Navigation
-
Googlebot's Entry Points: Understanding where and how Google enters your site is crucial. Tools like Screaming Frog and Jet Octopus help with this, but Jori advocates for manual approaches when tools are unavailable.
-
Sitemap and Backlink Mapping: Utilize tools to track how Googlebot navigates through the site from internal links and backlinks, identifying content hubs and dead zones.
Tools and Techniques
-
Screaming Frog: Great for analyzing logs and crawl behavior but can be overwhelming for beginners.
-
Jet Octopus: More sophisticated and user-friendly, offering CDN integration and template analysis for better insights.
-
Manual and App Scripts: Jori explains how to automate certain tasks, like downloading crawl data, using Google App Scripts and ChatGPT, sharing her custom-built tools for specific tasks.
Visualizing Crawl Behavior for Clients
-
Heat Mapping Entry Points: Jori demonstrates a heat-mapping approach to identify high-value or problematic entry points on a site.
-
Crawl vs. Index Visualization: Creating simple visuals can help explain the relationship between crawling activity and actual indexation, which is key to gaining stakeholder buy-in.
Key Takeaways
-
Actionable Crawling Strategies: Whether using tools or manual methods, the goal is to ensure that Google can efficiently crawl and index the most critical pages on your website.
-
Measuring Success: Jori highlights the importance of setting specific goals for crawl rates and indexation, then adjusting strategies based on real-time insights.
-
Lazy but Smart Automation: Jori humorously admits to preferring "lazy" methods, using automation to streamline repetitive tasks and maximize impact with minimal manual effort.
Video: https://www.youtube.com/watch?v=5N3-H5wcjV8
When is cloaking a good idea?
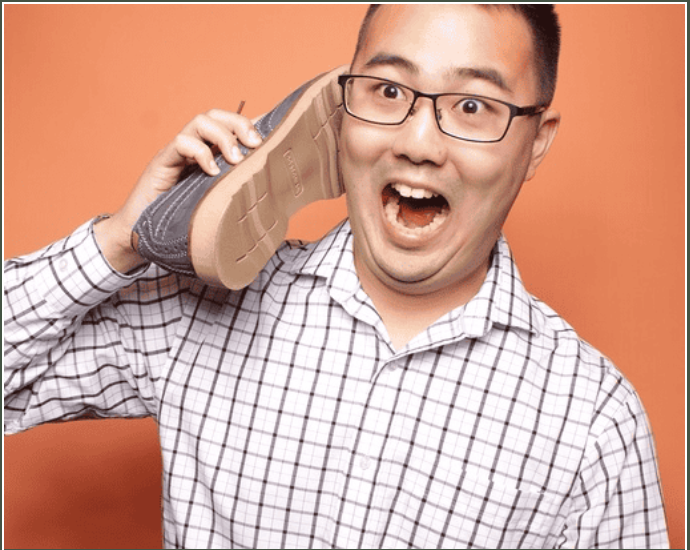
Summary Highlights:
Definition of Cloaking
-
Cloaking, in SEO terms, involves presenting different content to search engines than to users with the intent to manipulate rankings and mislead users.
-
Google's stance: Cloaking to mislead users or manipulate search rankings is against Google's guidelines and can lead to penalties.
Why Cloaking Might Be Considered
Victor shared real-world examples where cloaking (or a form of it) helped businesses manage critical issues like GDPR compliance and reduce unnecessary load on search engines:
-
GDPR and Analytics Consent: Many users, particularly in countries like Germany, deny cookie consent, breaking analytics tracking and attribution. Victor discussed a workaround where parameters were added via JavaScript to maintain last-touch attribution.
-
Reducing Crawl Load: When parameters started showing up on external sites, duplicate content was generated, and Google was wasting crawl resources. The solution was to block Googlebot from executing the JavaScript responsible for appending parameters, essentially cloaking Googlebot from seeing unnecessary data.
Strategic Cloaking for Efficiency
Victor emphasized that cloaking isn’t always malicious and can be a good idea in certain situations:
-
Blocking Unnecessary Data for Bots: For example, Googlebot doesn't need to see non-rendering scripts like ad tracking, heat maps, or personalization scripts. This could reduce server load and improve crawl efficiency.
-
Edge Computing: Serving different content, such as optimized images based on the user’s device (but not for Googlebot), can improve Core Web Vitals and user experience without affecting how Google indexes the page.
-
Dynamic Rendering: Personalization for users (based on cookies or location) doesn’t need to be served to Googlebot, so cloaking bots from personalized content makes sense when done correctly.
Practical Examples
-
Cloaking Meta Data: Blocking Googlebot from loading open graph data, schema, and other meta tags that aren’t necessary for users but are critical for search engines can improve load times and reduce unnecessary data transfers.
-
Reducing Parameter Handling: Victor suggests avoiding the unnecessary crawling of URLs with parameters and automatically redirecting to parameterless URLs with a 301 status. This saves Google's resources and ensures better content indexing.
When Is Cloaking a Good Idea?
Cloaking can be ethical and beneficial when:
-
It doesn't mislead users and delivers the same essential content to both users and search engines.
-
It saves resources, such as reducing server costs, improving Core Web Vitals, or achieving sustainability goals by reducing unnecessary data transfer.
-
It respects personalization, where cloaking helps deliver personalized content to users while ensuring search engines see consistent, relevant content.
Avoiding Risks
Victor repeatedly emphasized the caveats and risks:
-
If caught, cloaking can lead to manual penalties from Google.
-
Careful implementation is necessary to ensure the site remains compliant and the intent isn't to manipulate rankings but rather improve user experience and resource efficiency.
Video: https://www.youtube.com/watch?v=JW2hJB5TzEk
Microsoft Bing - SEO for the AI era
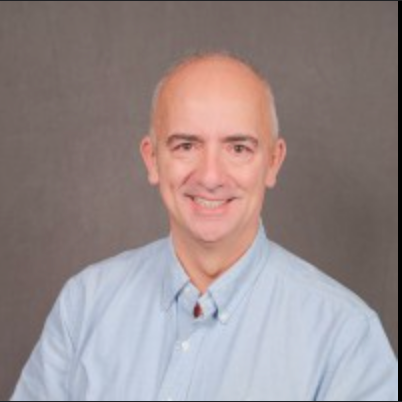
Summary Highlights:
Overview of Bing’s Mission and Reach
-
Bing’s mission is to create the world’s best index by downloading, processing, and surfacing content in Bing search results, AI-based experiences, and other services like news and advertising.
-
Bing’s reach goes beyond traditional search and powers experiences for companies like Yahoo, Meta, and even OpenAI. It is also integrated into Windows search and other platforms, often unnoticed in analytics due to the absence of a referrer.
The AI Revolution in Search
-
The rise of AI innovation has transformed how search engines function. In 2023, Bing introduced Copilot, an AI-augmented experience that allows users to have deeper interactions with search queries through chat-like experiences, improving user engagement.
-
Users now experience two kinds of search interactions:Navigational queries: Where the user knows exactly what they want, and the search engine tries to minimize the time between the query and finding the right result.Exploratory queries: Where the user isn’t entirely sure what they are looking for, leading to interactions with AI to discover the best results.
AI’s Impact on SEO
-
AI does not kill SEO; it diversifies it. AI-based experiences, like Bing’s Copilot or tools like OpenAI and Perplexity, are driving new types of traffic to websites.
-
High-quality traffic from AI: AI-driven clicks are often more qualified than regular search clicks, as they lead to better conversions, such as event registrations or purchases. SEO practitioners should focus not only on clicks but on conversion metrics to demonstrate value.
Bing’s New Features and Tools
-
Bing Generated Search: A new way of mixing AI into search results, providing users with a rich, magazine-like experience that anticipates what they might want based on previous interactions.
-
Deep Search: A slow but precise search function using LLMs (Large Language Models) to deeply process content and generate high-quality results.
-
Bing Webmaster Tools:A new Copilot assistant within Bing Webmaster Tools offers insights and support based on your site’s data, providing personalized recommendations.The Top Recommendations feature alerts webmasters to critical SEO issues such as missing H1 tags or meta descriptions, helping them improve their site’s performance.The Search Performance report has been extended to show 16 months of data, including traffic from both search and AI experiences.
IndexNow Protocol
-
IndexNow is a key focus for Bing, allowing webmasters to notify search engines when content has been added, updated, or deleted, reducing unnecessary crawling and improving indexing efficiency.
-
Adoption of IndexNow: Already supported by major players like Yahoo, eBay, and Cloudflare, IndexNow is a protocol that all search engines can benefit from. It ensures timely updates for content, such as price changes or video view counts, which are crucial for ranking and relevance.
-
Bing Webmaster Tools now shows insights on how well your site is using IndexNow, with an emphasis on deleting outdated URLs and updating content as it evolves.
Future Trends and Final Thoughts
-
AI in SEO: AI content generation is here to stay, and the best results come from a balance of AI and human insight. Take control of your crawl data and optimize for both SEO and AI-driven traffic.
-
Focus on conversions: Conversion rate is more important than the sheer number of clicks. AI-driven clicks often convert better, so track these metrics carefully.
-
Adopting IndexNow: IndexNow is crucial for keeping content fresh and relevant in search engines. Its adoption by more major platforms is expected to grow in the coming years.
Video: https://www.youtube.com/@techseoconnect/streams
How to incorporate machine learning into your SEO day-to-day
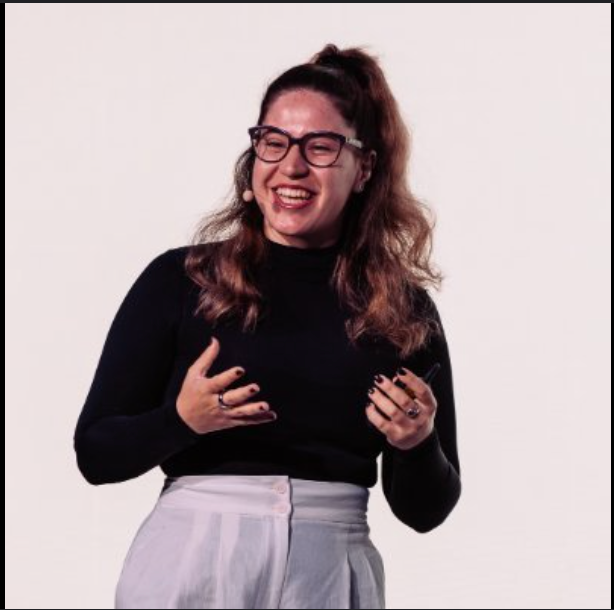
Summary Highlights:
What You Need to Start with Machine Learning
1. Task Characteristics:
- Supervised learning (e.g., regression and classification) vs. unsupervised learning (e.g., clustering and dimensionality reduction).
- Decide whether your task requires labeled data (supervised learning) or if you're exploring without labels (unsupervised learning).
2. Data:
- Understand if your data is textual, numeric, image-based, or time-series. This influences your approach and choice of ML models.
3. Solution:
- The solution depends on how mission-critical your task is and how consistent or explainable you need the results to be.
- Supervised learning is preferable for tasks where you need consistency (e.g., automating metadata generation), whereas unsupervised learning is useful for exploration (e.g., clustering).
Machine Learning Models in SEO
Lazarina shared several practical use cases where machine learning can provide immediate value:
1. Text Classification:
- Using pre-trained models (like Google Natural Language API), you can classify pages into primary, secondary, and tertiary categories to improve internal linking, tagging, and content structuring.
- Why not ChatGPT? ChatGPT is not fine-tuned for these specific tasks, and its results are inconsistent. It's better to use tailored models like Google's API that give you precision scores.
2. Clustering:
- Helps group content or keywords based on shared characteristics, improving topic modeling and internal linking.
- Tools like LDA (Latent Dirichlet Allocation) and BERTopic can provide more advanced clustering for large websites.
3. Keyword Clustering:
- Dimensionality reduction to identify key terms or bi-grams within keywords or content. This is crucial for grouping keywords into clusters for SEO purposes.
- Google Colab templates can automate this process and help you organize keywords efficiently.
4. Entity Recognition:
- Entity extraction allows SEOs to identify key entities in content, whether it’s people, organizations, or other concepts. Tools like Google Cloud’s NLP API can give precise data on entity prominence and how they are referenced.
5. Fuzzy Matching:
- Used to match similar strings, this technique is useful for redirect mapping, competitor analysis, or detecting structured data patterns like FAQs.
6. Content Moderation:
- Content moderation models can help analyze whether your content is “Your Money or Your Life” (YMYL) and potentially problematic for ranking. This can also help with understanding competitor content and its alignment with YMYL standards.
7. Transcription for SEO:
- Transcribing video content (like YouTube videos or TikToks) into blogs or other text formats helps maximize content output across multiple channels. It’s particularly useful for content repurposing in large organizations.
8. Text-to-Text Transformation:
- This includes transforming blogs into social media posts, newsletters, or even generating product descriptions. LLMs (like GPT-3) can be highly effective for these tasks if you provide structured data to prevent hallucinations.
9. Use Cases for LLMs (ChatGPT)
- OpenAI’s GPT models work well for generating short social media posts, product descriptions based on structured data, and other similar tasks where flexibility is needed.
- These models are less reliable for precise tasks like entity recognition or text classification, where accuracy is key.
10. How to Implement Machine Learning in SEO
- Tools and Resources: Lazarina shared that there are beginner-friendly resources available, including Google Cloud APIs, Google Colab notebooks, and other templates that can simplify ML tasks.
- Community & Learning: Building a community around machine learning for SEO (like Lazarina’s ML for SEO community) can help share knowledge and provide templates to make the learning curve easier.
Key Takeaways:
1. Don’t aim for perfection: Use ML models to automate tasks and make work faster, not to replace human expertise.
2. Precision matters: Tools like Google Cloud's API outperform general-purpose models like ChatGPT in specialized tasks.
3. Integrate across teams: Machine learning can help bridge gaps between different teams, especially when dealing with large volumes of content, social media, and video transcriptions.
Automating Marketing Tasks With AI

Summary Highlights:
The Role of AI in Marketing
-
Generative AI is transforming marketing: The adoption of AI in marketing is growing exponentially, and industries like content marketing, SEO, and PR are experiencing some of the first disruptions. Tynski’s focus is on data journalism, content creation, and automation using large language models (LLMs) with human oversight.
-
Content creation will become nearly zero-cost: With AI's rapid advancements, we’re moving towards a future where content can be created at little to no cost, though the challenge remains in ensuring that the content provides unique value rather than being easily replicable by others.
AI Model Progression
-
AI models are advancing rapidly: Tynski highlights the growing capabilities of AI models, with tools like GPT-4 reaching near human-level performance in various domains, including reasoning and knowledge work.
-
Agentic frameworks: These frameworks allow AI to handle multitask workflows autonomously. Examples include GPT-4 and GPT-4 Turbo, which offer more sophisticated reasoning and can perform tasks that traditionally took humans weeks or months.
Key Applications for AI in Marketing
-
One Input, Many Outputs:
-
Content Generation from Sources of Truth:
-
Advanced SEO Tools:
-
Multimedia and Social Media Automation:
-
FAQ and Schema Automation:
-
Retrieval-Augmented Generation (RAG):
Impacts of AI on Marketing and SEO
-
Decreased organic traffic: As users turn to AI tools (e.g., ChatGPT) to answer their questions directly, click-through rates and organic traffic to websites may decline.
-
Dead Internet Theory: Tynski discusses the idea that AI-generated content could overwhelm human-generated content, diluting the quality and reliability of the web. Marketers will need to adapt by producing high-quality, data-driven content.
-
New Content Standards: The future of SEO will prioritize data-backed, original content. AI enables the creation of data journalism at scale, which will be the gold standard for content that ranks well.
Future Trends in AI Marketing
-
Real-time AI Agents: AI will evolve into autonomous team members, executing complex workflows with minimal human oversight.
-
Larger Context Windows: With the ability to process larger amounts of data at once, AI models will be able to tackle even more comprehensive tasks, potentially replacing traditional search engines.
-
Generative Video and Audio: Tynski mentions advancements in AI-generated video and audio, including real-time podcast creation, making multimedia content creation more accessible.
Key Automation Pipelines and Scripts
-
Google Colab & Python: Tynski emphasizes the power of AI for automating tasks with Google Colab and Python scripts. She shares 25 automation scripts ( Link will be added Soon ) that marketers can use for SEO, content generation, keyword research, and more, making these workflows faster and more scalable.
Video: https://www.youtube.com/watch?v=5YUiF7RYwHw
Accounting for Gaps in SEO Software
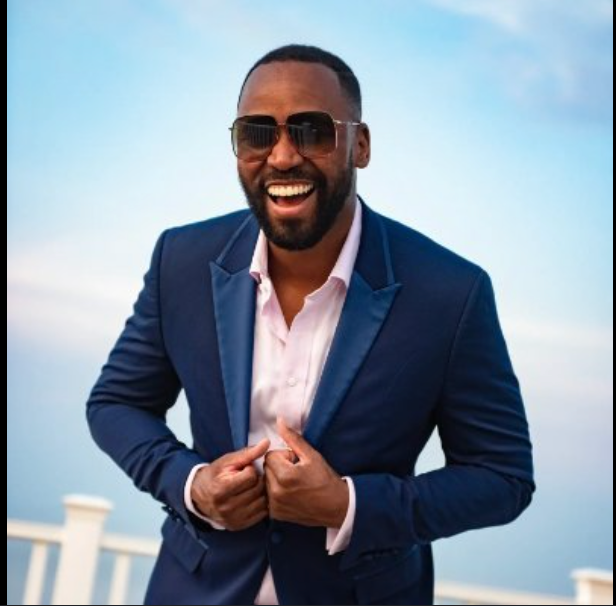
Summary Highlights:
SEO Software Gaps and Limitations
1. Current SEO Tools Miss the Mark: Many popular tools, particularly content optimization tools, still rely on outdated models. Tools that score content purely based on keywords (TF-IDF) and comparisons to the top 20 pages fall short because they fail to account for Google's shift to semantic search and contextual relevance.
- Google uses phrase-based indexing and other models that look at co-occurring keywords and how they relate across a broad set of queries. Tools that don’t account for this fail to optimize for what Google actually considers important.
2. How Google Truly Understands Content:
- Vector embeddings: Search engines like Google no longer just count keywords but use embeddings to map content in a multidimensional space where relevance is quantified based on proximity. This shift to semantic relevance allows Google to understand nuances and context, which is not fully captured by many SEO tools.
- Hybrid retrieval models: Google combines lexical matching (keywords) with semantic understanding (context), allowing pages to rank for terms without explicitly using them. This explains why some results might rank despite not having exact keyword matches in titles or H1s.
3. Dense Retrieval and AI Overviews:
- Google's ability to rank down to the sentence or paragraph level, scoring aspects of content separately, creates challenges for SEOs who rely on outdated metrics. Google uses multi-aspect dense retrieval, meaning that even fragments of a page could be relevant for ranking.
The Evolution of Search Algorithms
1. From TF-IDF to Word Embeddings:
- Traditional models like TF-IDF are based on counting word frequency, but with advancements like Word2Vec and later BERT, search engines can understand the meaning of words in context. This shift powers modern SEO strategies like topic modeling and relevance analysis.
- BERT vs. Word2Vec: While Word2Vec allowed for understanding relationships between words, BERT brought contextual understanding, allowing Google to differentiate between similar words used in different contexts (e.g., "bank" in "riverbank" vs. "financial bank").
2. Transformer Models and Generative AI:
- The rise of Transformer models (the "T" in BERT and ChatGPT) brought even more advanced language understanding. These models allow Google to score and rank content based on deep semantic relationships rather than just surface-level keyword matching.
User Behavior and Ranking
1. Click Metrics and Behavior:
- Click data has long been a debated factor in SEO. Despite Google's public denials, leaked information (and DOJ testimony) confirms that user behavior, particularly click metrics like last longest click (users staying on a site without returning), significantly influences rankings.
- Reranking and Learning to Rank (LTR): Google adjusts rankings based on user behavior. Reranking algorithms monitor user engagement and satisfaction signals (clicks, hovers) to refine results.
2. The Role of Clickstream Data:
- King emphasizes that Google’s advantage is its vast clickstream data. Tools like SEMrush Trends and SimilarWeb provide a glimpse into this data, but no SEO tools fully harness the insights that Google has.
Embeddings and SEO Strategy
1. Embeddings for Sites and Authors:
- Google creates site-level embeddings, where the entire content of a site is represented as a single vector. This allows Google to understand what a site is about holistically, and determine how well individual pages align with that core topic.
- Author embeddings: Similarly, Google averages an author’s body of work, allowing the search engine to determine the relevance and trustworthiness of a piece of content based on the author’s established expertise.
2. Information Gain:
- Information Gain is a key factor in ranking. This metric assesses how much new, unique information a page provides compared to other pages on the same topic. SEO tools rarely measure this, though it’s a crucial aspect of search rankings.
- King provides a formula to calculate Information Gain using mutual information and entity extraction, allowing SEOs to measure how much novel content their pages offer.
Closing Gaps with Custom Tools
1. Developing Better SEO Metrics: King has developed several custom Python tools and Google Colabs to address the gaps in existing SEO software. These tools help compute:
- Site Focus Scores: Measure how well each page aligns with the overall focus of the website.
- Content Audits: Assess the potential of content for optimization using a composite metric called Content Potential Rating.
- Embeddings-based Audits: These tools analyze entire websites and individual pages to determine how semantically aligned they are with their core topics.
2. The Search Telemetry Project:
- King is working on the Search Telemetry Project, aiming to provide more comprehensive SEO metrics that align with what Google actually measures. This initiative will offer open-source data and community-driven tools to better track user behavior, content decay, and click metrics.
The Future of SEO Tools
1. Standardization:
- King advocates for standardizing SEO tools and data outputs. He points out the discrepancies between tools that provide different formats, making it difficult to switch platforms or integrate data. Standardizing would allow for more fluid use of SEO software across the industry.
2. Open-Source SEO Data:
- King proposes creating an open-source index of rankings, embeddings, and link data that the SEO community can access for free. Inspired by the Majestic model, he envisions a community-powered initiative that democratizes access to critical SEO data.
The Shift in Search Engines to Answer Engines
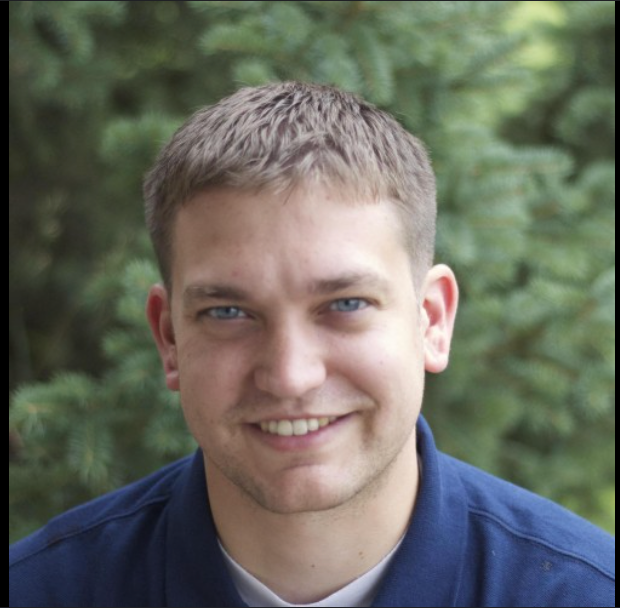
Summary Highlights:
-
Search engines are becoming answer engines: Instead of simply retrieving information, engines like Google and competitors such as ChatGPT and Copilot are shifting towards providing direct answers to users’ queries.
-
Increased access to powerful tools: With the rise of open-source models (like OpenAI and Google’s Vertex AI) and APIs for natural language processing and embeddings, SEOs now have more access to advanced tools that were previously hard to afford or access. Costs for tools like Google’s NLP API have decreased by as much as 99.9%, making large-scale applications more feasible.
Experimenting with AI and Embeddings for SEO Automation
-
Learning by building: Dan emphasizes the importance of jumping into projects to understand the power of AI and embeddings. One unique project he developed was a personalized chatbot, ChatGPT with Grandpa Hank, which used his grandfather’s journal entries, voice, and a language model to simulate a real-life conversation. This experiment helped him understand how tools like embeddings and voice cloning can be used in creative ways, and ultimately apply those concepts to SEO.
Addressing Language Model Limitations
-
Handling math and accuracy issues: One major limitation of large language models is their inability to handle tasks like counting characters or ensuring factual accuracy. Dan suggests two major solutions:Function calls: LLMs can call external functions (like calculators) to check character counts or perform specific tasks. This dramatically improves their performance.Explicit instructions and responses: To prevent LLMs from hallucinating, they need clear instructions. For instance, if the AI is unsure of an answer, it should be told to say “I don’t know.” By providing more structured instructions, error rates drop significantly.
Automating SEO Tasks with Large Language Models and Embeddings
-
Embedding databases: To ensure that large language models have access to accurate data, it’s crucial to embed data into a vector database (using text embeddings). These embeddings allow the model to retrieve the most semantically relevant information, similar to how search engines operate.
-
Semantic content evaluation: Dan introduced a Chrome extension his team built that scores content using semantic similarity scores, powered by Google’s Vertex AI. This tool analyzes the content on a page, scores it based on a target keyword, and highlights areas that need improvement using heatmaps. It allows SEOs to see which content is most relevant to the keyword and where improvements can be made before making any changes live.
Practical Examples of Automated SEO Tools
-
Identifying content freshness and search intent: Automated tools can quickly scan SERPs to determine the freshness of content and assess user intent.
-
Finding content gaps: By scraping competitor websites, tools can help identify content gaps that your site may not be covering. Dan recommends using JSON as the structure for passing data to language models since they handle it well.
-
Discovering Information Gain opportunities: Tools can identify the common facts across competing sites and highlight missing or unique information that can help improve your content.
-
Optimizing internal linking opportunities: By analyzing the semantic similarity between paragraphs on different pages, SEOs can identify potential internal linking opportunities.
-
Analyzing image attributes: Tools can analyze the image tags on a site and identify any missing or unoptimized alt text or filenames.
-
Topical authority assessments: Automated tools can compare your site's content against competitors, analyzing which site has more authority based on semantic relevance to the target topics.
Automated SEO Tool: Barracuda
-
Barracuda: This is an internal tool developed by Dan’s team at Go Fish Digital that automates the collection of data across competing websites, analyzes it, and generates SEO insights. Barracuda can gather and analyze data that would take four hours manually in just four minutes, significantly improving SEO team efficiency.
Conclusion
-
Automation is now accessible: The rise of powerful, affordable tools means SEOs can automate many tasks that were previously manual. Automated tools can now handle data gathering, content scoring, and content recommendations at scale, freeing up SEOs to focus on higher-level strategies.
-
AI + Me: how we built a GSC Bulk Export data pipeline

Summary Highlights:
The Vision: Merging Tools for GSC Data Insights
Noah aimed to create a tool that could combine GSC Bulk Data Export with his Branch Explorer product. The idea was to build a system that could handle GSC data more efficiently at scale, providing deeper insights into search data. The tool would allow SEOs to see GSC data across multiple dimensions and search types—something not possible with standard tools.
What is the GSC Bulk Data Export?
-
Bulk Export Overview: Google Search Console's Bulk Export pushes GSC data directly to BigQuery, giving users access to full datasets without the row limitations of the API.
-
Three Tables: The data export contains three tables:
-
Detailed Fields: The export provides fields like anonymized queries, device types, search appearances (e.g., AMP, videos, jobs listings), and Boolean fields for search features (e.g., Top Stories, shopping).
-
No Row Limits: Unlike the API, which is limited to 50,000 rows per day, Bulk Export has no limits, making it ideal for large-scale data needs, including multiple search types like Discover, Google News, Images, and Video.
Why Use Bulk Export for Small Sites?
Noah emphasized that even small sites could benefit from Bulk Export by gaining insights into anonymized queries, search appearances, and other granular data, previously unavailable.
Building with AI: The Journey and Challenges
Noah built the tool entirely using AI (such as ChatGPT, Claude, and other LLMs), and his journey was full of lessons learned:
-
AI's Capabilities and Limitations:
-
Iterative Development Process:
-
Effective Tools for AI-Assisted Development:
Lessons Learned from the Bulk Export Data Pipeline
-
No Backfill: GSC Bulk Export does not provide historical data, meaning you only get data from the moment you activate the export.
-
Costs: Though exporting data to BigQuery can seem expensive, Noah built a calculator to estimate daily data costs based on partition size and other factors. He used AI to build this tool.
-
Advanced Use Cases: The bulk export allows for advanced segmentation, tracking search appearances, and building custom data pipelines that can improve visibility for sites of any size.
Insights into AI Development Pitfalls
-
Natural Language Issues: AI models often misunderstood coding tasks, adding unnecessary language elements into code.
-
Iteration and Errors: Noah demonstrated that tools like ChatGPT and Claude often fail to resolve errors properly, leading to a frustrating cycle of back-and-forth prompts.
-
Custom GPTs: He built various custom GPTs to assist in coding, including one for Google Cloud Stack, WordPress, and AppScript. Each of these tools helped speed up repetitive tasks and improve productivity.
The Final Tool: Branch Explorer with Bulk Data Integration
-
Branch Explorer: A Looker Studio-based visualization tool that integrates with GSC Bulk Export, allowing users to explore search data in ways that are not possible with the standard GSC interface. Features include segmentation by subdirectories, UTM parameters, and device types, as well as analysis at the funnel stage (e.g., brand vs. non-brand queries).
-
Tool Availability: Noah shared a QR code and URL for users to access the tool and set up their own data pipelines.
Conclusion
Noah’s journey with AI and GSC Bulk Export highlights the potential—and the challenges—of building advanced SEO tools with AI. While AI can assist in speeding up some development tasks, it still has limitations, particularly when managing complex tasks or ensuring code accuracy. Nevertheless, with persistence and the right tools, SEOs can leverage Bulk Export data to unlock new insights and efficiencies.
Video: https://www.youtube.com/watch?v=77smtS14x3M
Can GSC Be the Source of SEO Decisions?

Summary Highlights:
1. The Three Data Pillars for Technical SEO:
Serge emphasizes that for technical SEO, three main data sources are crucial:
-
Crawlers: Provide insights into the site structure, internal linking, and other on-site factors.
-
Log Files: Show how Googlebot perceives your website and help in tracking crawl budgets.
-
Google Search Console (GSC): Acts as the funnel that connects crawling, impressions, and organic clicks.
2. GSC: Interface vs. API:
-
User Interface Limitations: The GSC web interface only allows for the export of limited data (thousands of rows).
-
GSC API: For larger websites, using the API is essential to handle massive amounts of data. While the API offers basic functionality (queries, site analytics, etc.), there are still significant gaps in terms of comprehensive data access.
3. Challenges of GSC Data Accuracy:
Serge warns against fully trusting GSC data, stating that critical thinking is essential when interpreting the results. Even if you pull data via the API, discrepancies can arise, particularly with longtail queries and anonymized queries.
-
Verification of Data: Always verify your results when working with GSC data. Serge shows examples where GSC inaccurately reports impressions and clicks when broken down by page and query, leading to confusion, especially for longtail pages that are crucial for technical SEO optimization.
-
Incomplete Data: GSC can significantly underreport data for longtail pages, which are vital for technical SEO growth.
4. Issues with Aggregation and Data Discrepancies:
-
Aggregation Types: Serge highlights the importance of understanding how GSC aggregates data. For example, when aggregating by property vs. page, you can get different impressions and clicks, which can be misleading when making SEO decisions.
-
Mobile and Desktop Data Mismatch: Sometimes, impressions and clicks do not align when broken down by device type, and even adding up mobile and desktop queries doesn’t always match the total, leading to inconsistencies.
5. Anonymized Queries and Pages:
-
Google often anonymizes certain queries, and these can account for a large portion of the data, especially for longtail queries.
-
Serge questions why pages are also anonymized in GSC and why certain pages with multiple impressions are not reported accurately.
6. Subfolder Strategy to Improve GSC Data:
-
To better manage GSC data, Serge recommends adding subfolders as properties within GSC. This method helps break down the data more effectively and reduces the chance of data discrepancies.
-
Considerations: While adding subfolders is a quick fix, managing the data afterward becomes complex due to duplication across the main property and subfolders. Serge warns against using AI for merging this data due to the high risk of errors.
7. Subfolder Implementation Results:
-
By implementing subfolders, one client increased the number of pages tracked in GSC from 80K to over 4 million, showing a significant boost in data granularity.
-
However, even with this improvement, there are still gaps in capturing all longtail queries and impressions, especially for enterprise-level websites.
8. Challenges of GSC Bulk Data Export:
-
While GSC Bulk Data Export allows access to a larger dataset compared to the API, Serge has found limited use cases in practice. The security and configuration challenges, especially with large enterprise sites, make it less appealing for widespread adoption.
-
Bulk Export is more complex to integrate and use compared to the API, particularly when dealing with Google Cloud Storage setups and security reviews.
9. Conclusion:
-
No Perfect Solution: Serge concludes that while GSC is an invaluable tool for SEOs, it has its limitations, particularly for longtail queries and large websites.
-
Be Wary of GSC Data: When working with GSC, especially for making strategic decisions, always be aware that the data might not be complete or accurate. This is particularly important when dealing with content pruning or longtail optimization.
-
API as a Practical Choice: Despite the flaws, GSC API remains a practical solution for large-scale sites due to its ease of use and integration with other SEO tools.
In the end, Serge's message is clear: GSC can be a valuable source of SEO insights, but it cannot be the sole or fully trusted source due to various data limitations and inconsistencies. It’s crucial to cross-verify GSC data with other sources and always apply critical thinking when interpreting the results.
Video: https://www.youtube.com/watch?v=XMCLoeILGp4
BigQuery for SEOs

Summary Highlights:
Sam Torres, a former developer turned SEO expert, discussed how BigQuery can help SEOs manage and analyze vast amounts of data efficiently. Her presentation emphasized the power of BigQuery to address limitations in Google Search Console (GSC) and Google Analytics 4 (GA4), especially for large-scale data storage and reporting needs.
Key Themes and Takeaways:
1. BigQuery as a Solution for Data Storage and Scalability:
-
Google Search Console (GSC) Limitations: SEOs are limited by GSC’s 16-month data retention window and the delays associated with data in Looker Studio (formerly Data Studio). BigQuery provides a scalable, unsampled data storage solution to avoid these issues.
-
Why BigQuery?: BigQuery allows for storage of vast amounts of data (unsampled), providing flexibility in accessing data beyond standard GSC limits. It integrates with GA4, GSC, and other marketing platforms, making it ideal for agencies managing data across multiple sources.
2. Understanding BigQuery Pricing:
-
Two Main Costs:Storage Costs: BigQuery offers 10 GB of free storage, which is often sufficient for storing large amounts of SEO-related data (like GSC data). Storage is very affordable beyond the free tier.Computation Costs: BigQuery offers 1 TB of free queries each month, but exceeding this limit can lead to higher costs. Sam warned that inefficient queries can quickly increase costs, so it’s essential to optimize queries.
-
Cost Control: Set budget alerts to monitor spending and avoid unexpected costs. Even for large clients, costs typically remain under $10 per month for SEO data queries.
3. How to Set Up BigQuery with GSC and GA4:
-
Bulk Export from GSC: Google offers bulk data export for GSC, which allows for easy storage of GSC data in BigQuery. Sam recommended setting up a project in Google Cloud Console and linking GSC to BigQuery via API.
-
Setting Up for GA4: Similar to GSC, GA4 data can be streamed to BigQuery. Sam advised selecting only the most relevant events to limit the number of events per day (max 1 million), reducing unnecessary data storage and computation costs.
4. Navigating BigQuery and Structuring Queries:
-
Two Tables in GSC Bulk Export:Site-level Impressions: Aggregated data for the entire website.URL-level Impressions: Detailed data for individual URLs, including SERP features.
-
GA4 Tables: GA4 generates a table for every day, with events nested within the table structure. Each event becomes the fundamental unit of analysis.
-
Using SQL in BigQuery: BigQuery uses SQL (Structured Query Language) to interact with the data. SQL is a well-documented, old language that is widely supported, making it easier to use for querying SEO data.
5. Tools to Simplify SQL and Queries:
-
Query Tools: Sam mentioned tools like GA4 for SQL, which offer user-friendly interfaces for building SQL queries without needing to manually write code. These tools allow you to select metrics and build complex queries easily.
-
SQL Basics: Queries in BigQuery follow a typical structure:SELECT – Specify the data to retrieve (e.g., clicks, impressions).FROM – Define the data source (e.g., GSC URL table).WHERE – Filter the data (e.g., only U.S. data or a specific date range).ORDER BY – Organize the data output (e.g., sort by most clicks).
6. Creating Efficient Data Workflows with Aggregate Tables:
-
What Are Aggregate Tables?: Sam introduced the concept of "aggregate tables," which are pre-processed tables that summarize raw data. These tables reduce the computation load for daily reporting.
-
Why Use Aggregate Tables?: Connecting Looker Studio directly to BigQuery’s raw data tables can result in high computation costs, as queries run every time a report is refreshed. Instead, Sam recommended using aggregate tables to store pre-queried data. These tables are updated once per day, saving costs and reducing query times.
7. Automating Data Updates and Optimizing Reports:
-
Scheduling Jobs in BigQuery: Sam demonstrated how to schedule SQL queries in BigQuery to update aggregate tables automatically. She advised scheduling these updates daily, ensuring fresh data without overloading BigQuery or Looker Studio.
-
Efficient Reporting with Looker Studio: By connecting Looker Studio to the pre-processed tables in BigQuery (instead of raw data), SEOs can reduce lag times and computation costs when generating reports. This is especially useful for clients who frequently refresh reports.
8. Measurement Plans and Use Cases:
-
Measurement Plan: Sam recommended using a measurement plan to guide which data to track and how to track it. This documentation is crucial for ensuring consistency, particularly when making updates to tracking setups.
-
Example Queries and Reports: Common queries include metrics like clicks, impressions, and query breakdowns by country, device, and channel. Sam emphasized starting small, building basic queries first, and gradually expanding the complexity as needed.
Conclusion:
BigQuery is a powerful tool for SEOs dealing with large datasets from GSC, GA4, and other platforms. It allows for scalable, cost-effective storage and analysis of SEO data, providing flexibility and overcoming limitations in tools like GSC. By using SQL and optimizing queries, SEOs can reduce costs and improve reporting efficiency. However, it’s essential to set up BigQuery correctly, manage costs, and use tools to simplify querying and data management.
Sam’s advice to the audience: Start small, be mindful of costs, and leverage aggregate tables to optimize data reporting.
What I learned from auditing over 1,000,000 websites
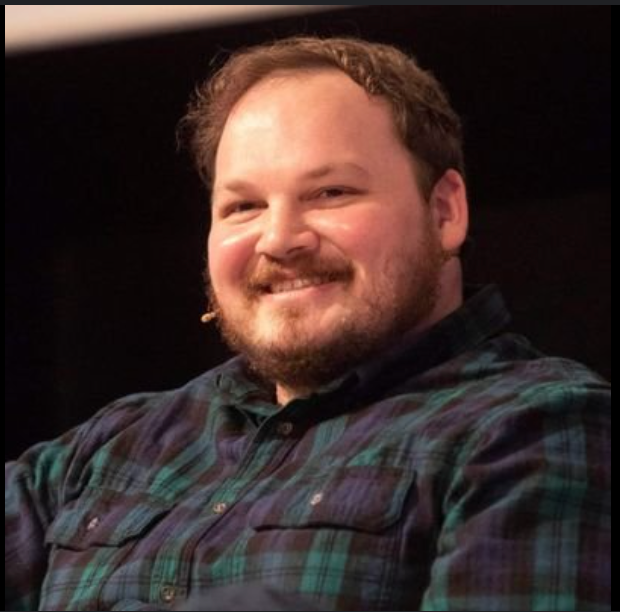
Patrick Stox, an experienced SEO professional, shared insights from auditing over 1 million websites. His talk provided a blend of practical advice, the importance of prioritizing SEO fixes, and new advancements in automation and AI for technical SEO.
Key Themes and Takeaways:
1. The Web is Messy:
-
Stox emphasized that websites are often a mess, with many issues popping up during audits. However, just because something is flagged as an issue doesn’t mean it’s critical to fix.
-
He shared the concept of prioritization in SEO fixes, using an impact-effort matrix to determine what is truly worth the time and cost to fix, considering the ROI.
2. Not All Issues Are Critical:
-
Broken Pages: If no one visits a broken page, does it really matter? If a broken page has no traffic or links pointing to it, it may not be worth the effort to fix it.
-
Multiple H1 Tags: Multiple H1s are often flagged as an issue in audits, but as long as the tags are relevant, it’s not a significant problem.
-
Broken Images: Similarly, if an image is broken on a page no one visits, fixing it may not be a priority.
-
Duplicate Content: According to Stox, about 60% of the web is duplicate content. Google is adept at handling duplicate content and canonicalizing pages correctly, so it’s usually not a major concern.
3. SEOs Sometimes Over-Prioritize Certain Issues:
-
Orphan Pages: Pages that are not linked internally are often highlighted in audits. However, if the site owner didn’t consider the page important enough to link to, it may not need to be addressed.
-
Redirect Chains: While redirect chains are flagged frequently, Stox noted that unless they exceed five hops, they usually have little impact and don’t warrant immediate action.
4. Focusing on ROI is Key:
-
Fixing every flagged issue can be a waste of resources. Stox recommended focusing on "easy wins" that offer high impact with minimal effort. He encouraged SEOs to focus on fixes that drive value, like addressing pages that aren’t indexed or optimizing internal linking structures.
5. Specific Issues Worth Addressing:
-
Alt Attributes: While alt text has minimal impact on SEO, it is important for accessibility and legal compliance in some regions.
-
Redirects: Retaining redirects is essential to maintain link equity from previous content. Stox mentioned the importance of automating redirects to avoid losing valuable backlinks.
-
Hreflang Implementation: Misconfigured hreflang tags can cause major issues, especially for international websites. He shared an example where improper hreflang implementation cost an e-commerce site $6.5 million per day in lost sales due to misdirected users.
6. The Rise of AI and Automation in SEO:
-
Stox discussed the rapid advancement of AI, particularly in automating technical SEO tasks. He shared his own experience using AI tools like ChatGPT to build scripts and tools, noting that tasks that once took him 12-14 hours now take as little as 45 minutes.
-
He mentioned IndexNow and Cloudflare Workers as examples of new technologies enabling faster indexing and page updates.
-
Automating SEO Fixes: Stox envisioned a future where nearly all technical SEO issues could be automated. He referenced a new tool, Patches, that automates tasks like generating meta descriptions or fixing no-indexed pages, saving SEOs hours of manual work.
7. Edge SEO and Serverless SEO:
-
Stox touched on Edge SEO, a concept coined by Dan Taylor, and how it allows SEOs to make real-time changes to code before it’s served to users or bots. This opens new possibilities for automating and optimizing websites without the need for traditional CMS changes.
Conclusion:
Patrick Stox’s presentation emphasized the need for prioritization in SEO, focusing on what will bring the most value rather than trying to fix everything. He highlighted the potential of AI and automation to streamline SEO tasks and encouraged SEOs to explore new tools and technologies that can make their work more efficient. His message to the audience was clear: focus on high-ROI tasks and embrace the future of AI-driven SEO.